Physics-Informed Neural Network Gravity Models
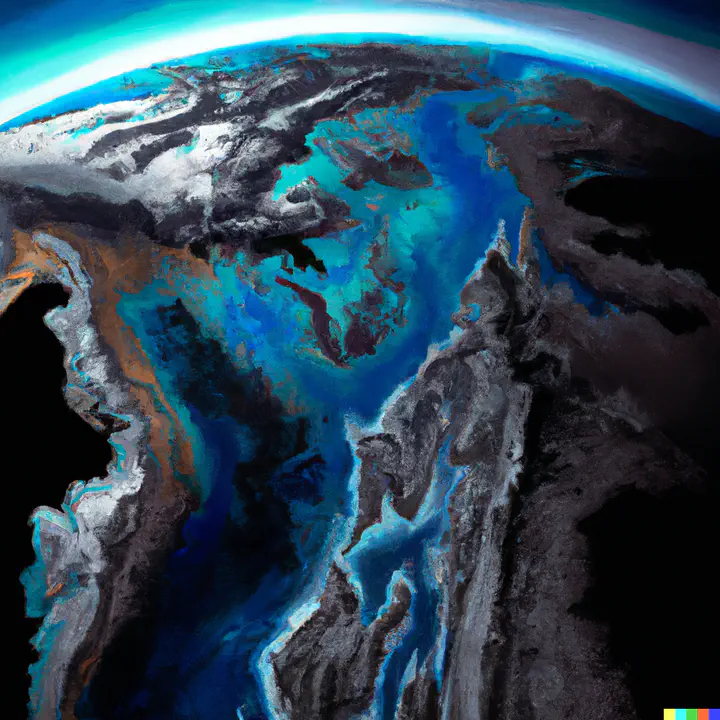
High-fidelity gravity models are critical data products for astrodynamicists, planetary scientists, and geodesists alike. These models enable the accurate prediction of spacecraft motion in exotic environments, the development of hypotheses of planetary formation and density profiles, and even inform climate scientists predicting glacial melt and sea-level rise. Despite the significance of these products, no universal model exists.
Currently there are many different ways to model a gravity field to high accuracy. One of the most common is the spherical harmonic gravity model, or the superposition of hundreds of thousands of harmonic basis functions to reconstruct a gravity field. While these spherical harmonic basis functions are powerful ways to capture large-scale gravitational perturbations like planetary oblateness, they becomes considerably less productive when attempting to represent local and discontinuous features like mountain ranges, tectonic plate boundaries, and hotspots. Moreover, the spherical harmonic gravity model entirely breaks down for irregularly shaped small-bodies like asteroids and comets. While alternative models exists, they each suffer from their own drawbacks ranging from large computational expenses, expensive memory footprints, or poor observability at high-altitudes.
The MLDS lab proposes a new type of gravity model that shifts attention away from pure analytics and toward data driven models. Specifically, by using Physics-Informed Neural Networks (PINNs), dynamicists can learn, rather than prescribe, unique basis functions to rapidly capture the idiosyncrasies of every gravity field. PINNs are neural networks that directly incorporate knowledge of the dynamical system into the training of traditional neural networks, granting greatly enhanced data efficiency, model accuracy, and robustness.
The MLDS lab continues to be the leader in developing PINN Gravity Models (PINN-GMs). These models are shown to be orders-of-magnitude more compact and efficient than state-of-the-art alternatives, making it a compelling alternative particularly in small-body mission contexts. Despite promising early results, further work remains to refine these models particularly for cislunar settings. This project continues these efforts with the development of PINN-GM IV.






