Shape Model Acquisition during Spacecraft Rendezvous using Neural Radiance Fields
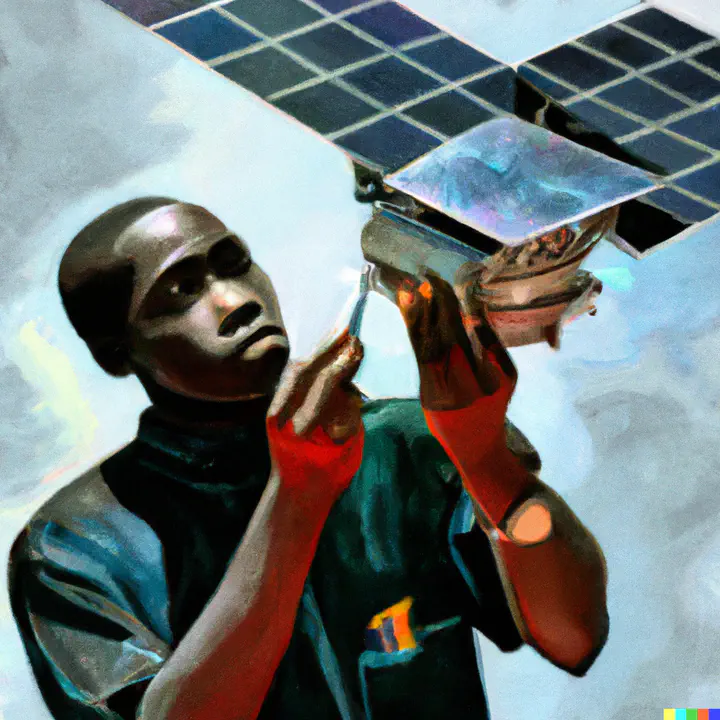
One of the most important responsibilities of satellite and spacecraft is to be able to construct a shape model of their target. These targets can include planets, asteroids, space debris, and other cooperative or combative satellites. In most cases, these objects are extremely difficult to resolve from the ground and require spacecraft to supply images in-situ that can be post-processed to construct the high-resolution shape models. These models, in turn, are used to inform navigation decisions, dynamics models, and potential areas for landing, contact, or science.
In addition to the shape-model generation process being cumbersome, these shape models can also consume large memory footprints, making them prohibitive to fly on-board spacecraft for feed-forward control purposes. To combat these challenges, this research proposes the use of neural radiance fields to construct more efficient shape models from sparse image data. Neural radiance fields (NeRFs) are a novel class of deep learning architectures that convert sparse raw images into 3D shape rendering of an object. This project encompasses using spacecraft visualization software to produce representative images of spacecraft in orbit, and designing NeRFs capable of accommodating the various lighting and imaging conditions found in deep space.