Orbital Mechanics Coordinate Discovery with Deep Learning
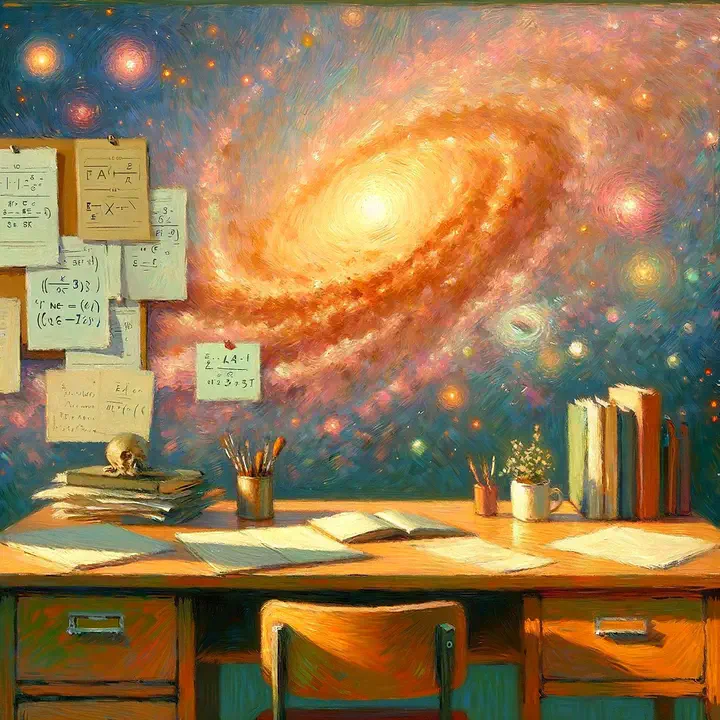
Orbital mechanics grow considerably more difficult when navigating spacecraft in settings with $N>2$ bodies. The seemingly small transition from the two-body problem into the three-body problem makes solutions that were once perfectly captured by six fixed elements into a problem whose dynamics are woefully difficult to characterize due to their chaotic nature. This increased complexity is unavoidable as all systems are $N > 2$. Take the cislunar environment for which many analytic efforts have been proposed to characterize satellite motion in this regime, identifying unstable and stable manifolds, the transfers between them, and families of orbits that can be leveraged for science and surveillance; however, the vast majority of these efforts rely on approximations and linearizations of the system to attain tractable analytics. This project proposes the use of deep learning to discover new latent coordinate descriptions of these chaotic environments using recent advances in scientific machine learning, and explores how these novel coordinate representations can be used for spacecraft guidance, navigation, and control.
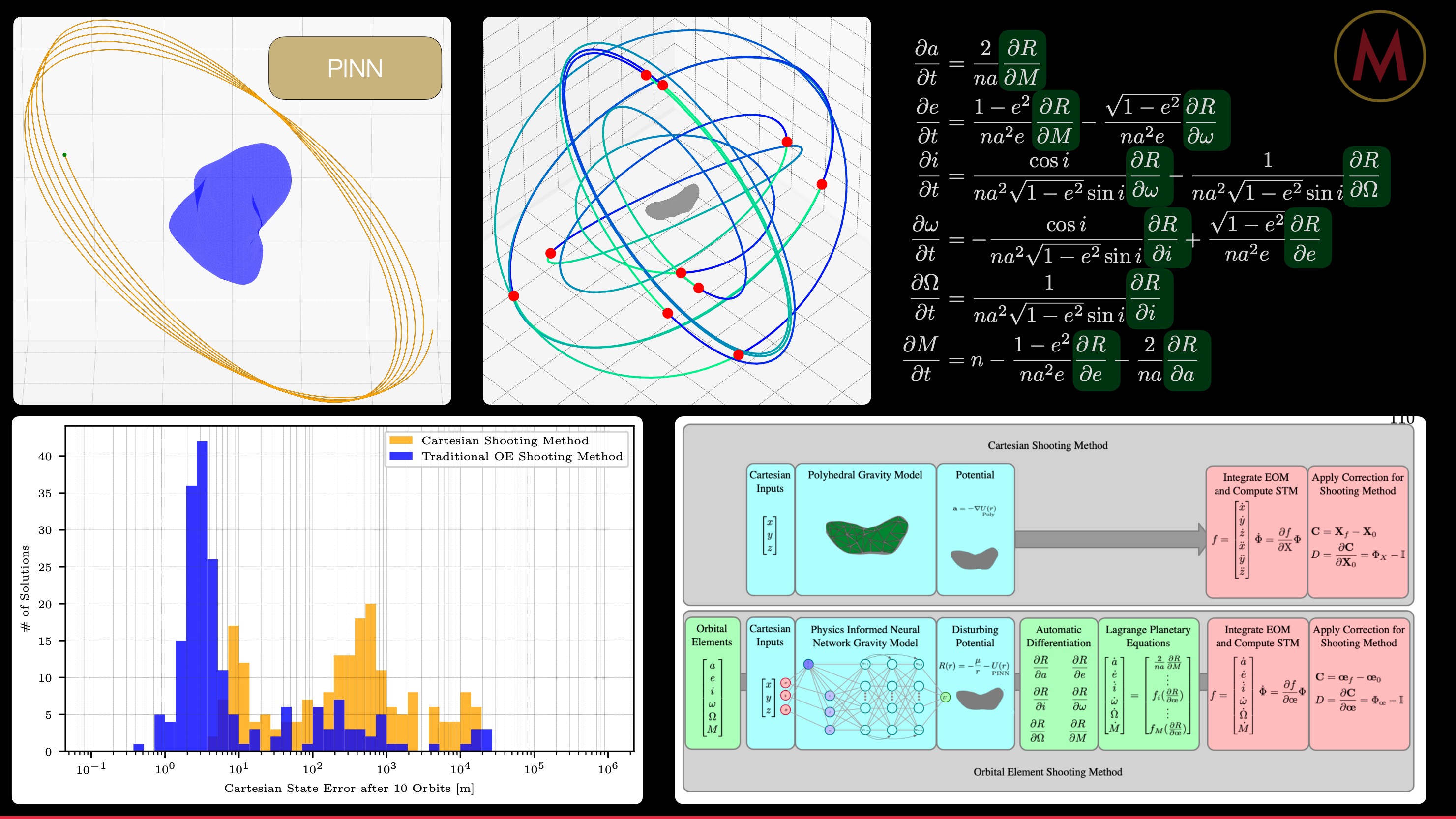
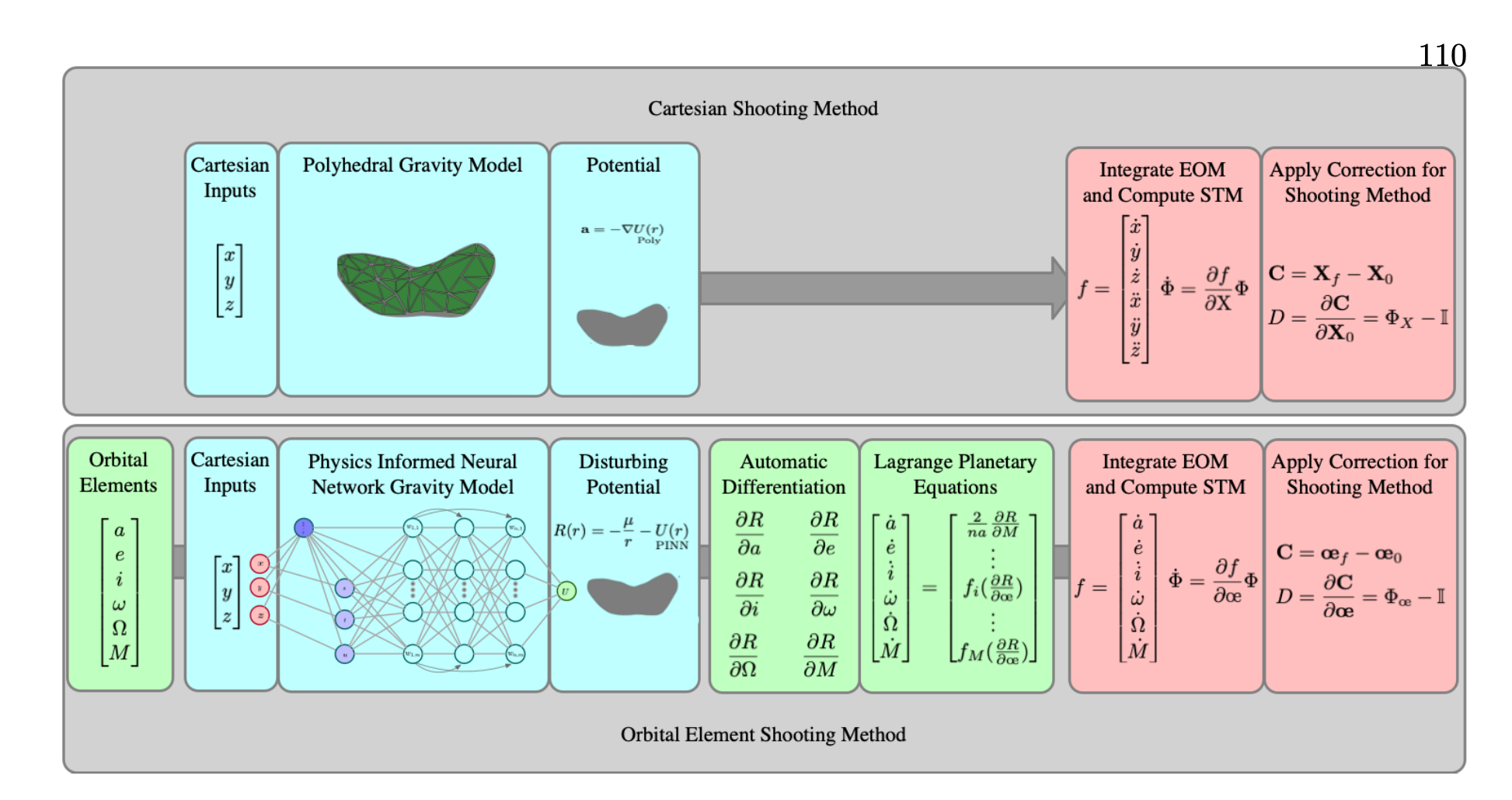
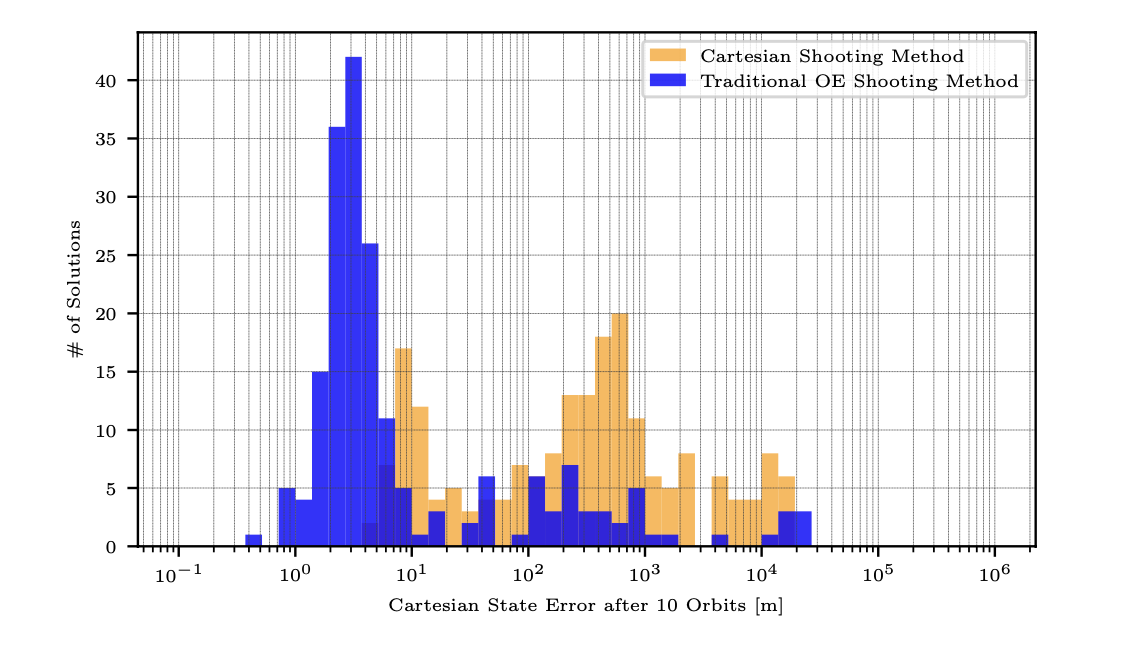
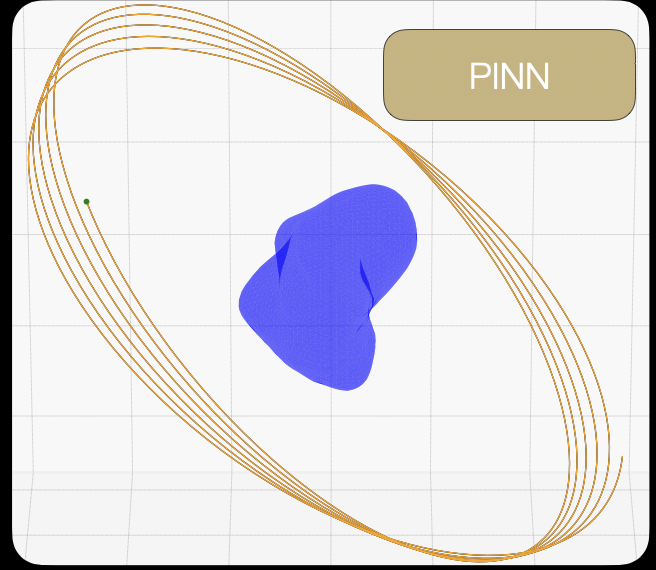
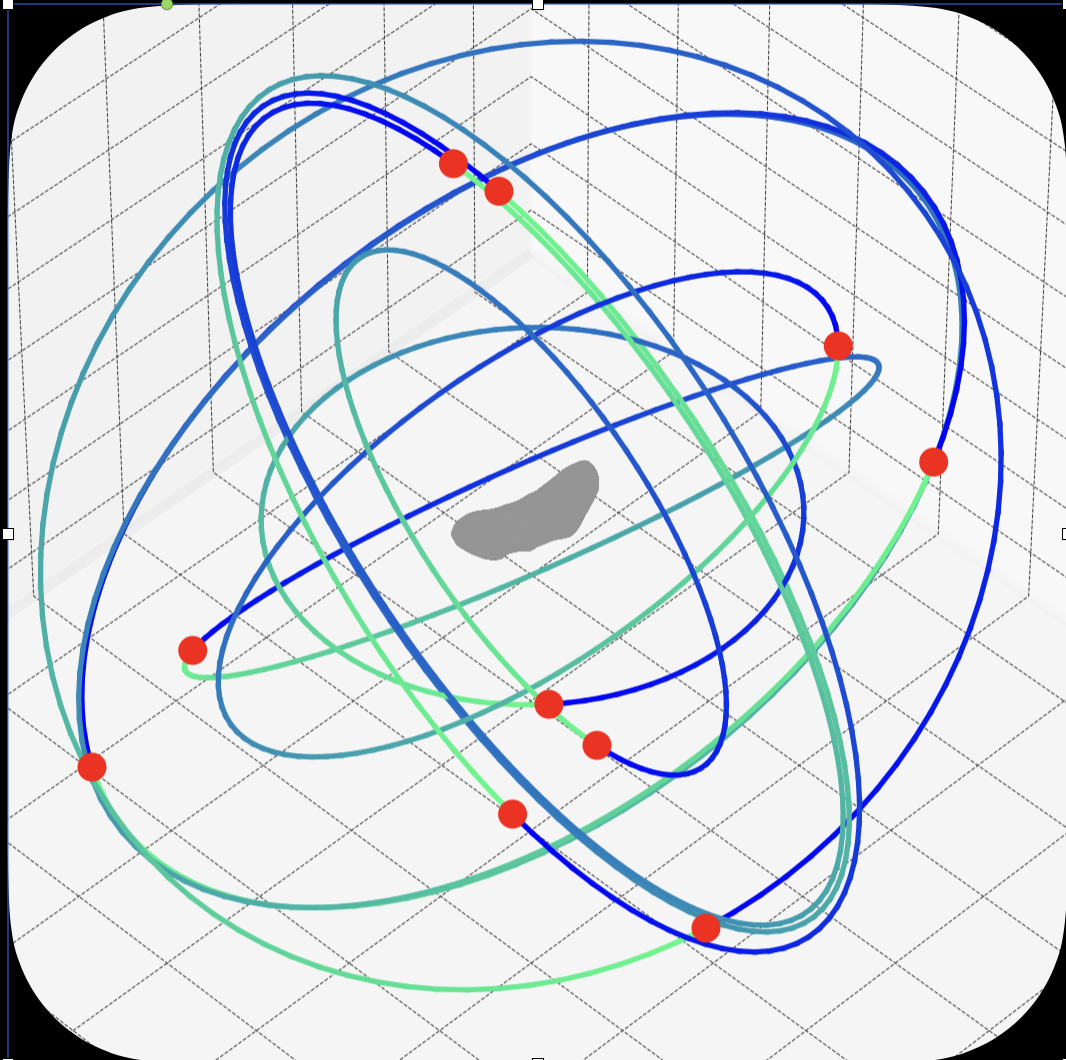